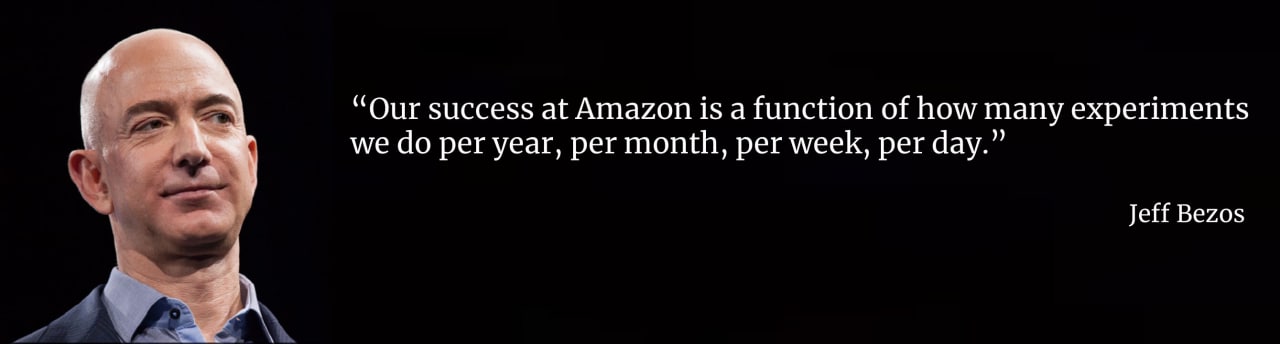
Booking.com’s loss from unsuccessful experiments is 2% of annual revenue. Let’s consider this as a benchmark. What revenue loss might a less experienced team have?
When you think about very costly experimentation mistakes, the first thing that comes to mind are things like “Buy” button bugs. But it’s not the most dangerous thing though, as it’s very evident, easily recognizable, and short-term.
The worst story I heard was of a 42% of annual revenue drop as a result of deploying a feature based on false positive experiment data.
We at Conversionrate.store have developed ~7200 A/B tests for 231 clients including Microsoft …. and 72% of our first 100 experiments had mistakes we realized only 8 months after starting A/B testing.
Here are 4 common problems related to experimentation that can dramatically decrease revenue or slow down the growth:
- Implementation of false-positive results
- No A/B testing at all for critical changes
- Direct revenue loss from underperforming variations
- Not maximizing the volume and velocity of experiments
All those issues are interconnected, so let’s go through 26 typical A/B testing mistakes that we see time and time again:
- Hypothesis is not focused on the main bottleneck
- Guessing reasons behind the main bottleneck
- Guessing how to fix the cause of the drop-off
- Holding the wrong metric like conversion-to-purchase as a goal
- Data tracking not at least 90-97% accurate
- No event mapping for all elements on A and B
- Testing more than one hypothesis per experiment
- Stopping the experiment only based on statistical significance
- No MDE and pre-test sample size planning
- No QA of alternative versions after experiment is launched and no monitoring of experiment session recordings
- No regression QA of the control version during an experiment
- No QA of experiment data tracking
- Not eliminating the “novelty effect”
- Implementation of false positive results
- No anomaly detection
- Outliers not cleaned up
- No preliminary A/A or A/A/B tests
- No analytics or tracking of long-term impact of implemented winning versions
- No in-depth post-test research and documentation of results
- Targeting irrelevant traffic segments together in one experiment
- Not checking for sample-ratio mismatch (SRM) for 100% of experiment traffic or all meaningful segments you want to compare
- Experiment data set not visualized
- Deploying winning versions to a different audience than in the experiment.
- Low experimentation velocity due to lack of in-house resources or absence of 100% dedicated experimentation teams
- Not leveraging parallel experiments when there is enough traffic
- Not speeding up experiment time with CUPED or similar techniques that leverage historical data on sensitivity of metrics.
Any alarm bells ringing? Even one mistake from the list can spoil experimentation results or slow down your growth rate.
Schedule a free A/B testing consultation where we can go through your experimentation process, discover its bottlenecks and consult on ways to maximize it’s volume, velocity and uplift.
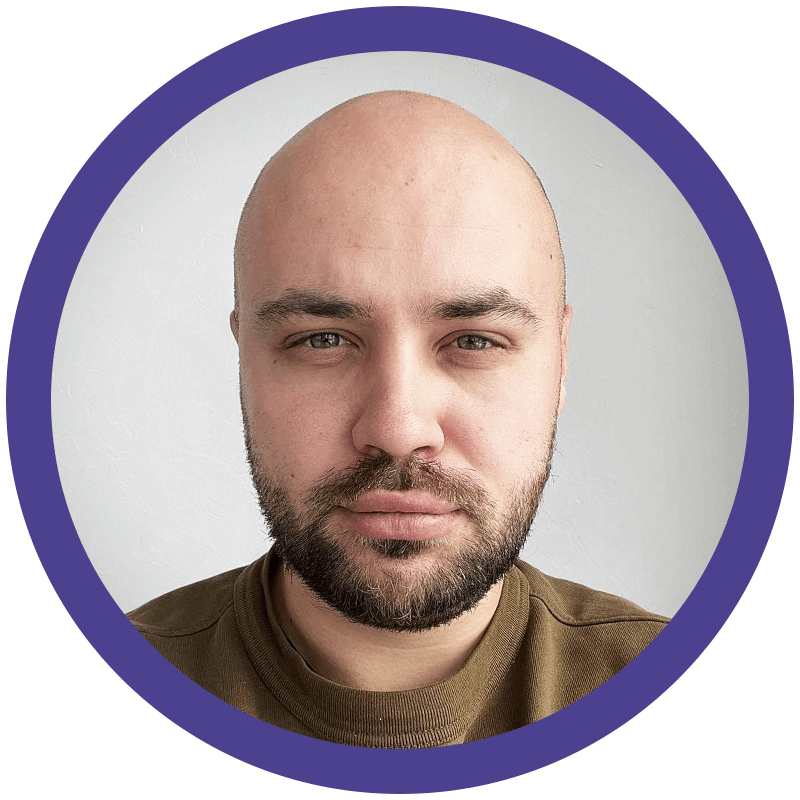
Glib Hodorovskiy, co-founder Conversionrate.store
Conversionrate.store is a performance-based funnel conversion rate optimization agency that worked with 3 NASDAQ-listed clients (Microsoft, GAIA, CarID).