How to create an effective CRO program that consistently results in more than 5% total revenue per user growth from a winning experiment?
If you ask to choose only one most critical factor of a conversion rate optimization program, that would be a depth of analytics and UX research plan. So that you back up your hypotheses with strong, statistically valid data on the actual reasons behind the main conversion barriers.
Michal Parizek, Growth Product Manager at Smartlook formulated that in a way more clear way:
Every CRO agency claims a depth of data behind hypotheses and CRO plan. And we are no exception! But what does “scientifically arriving at a hypothesis” actually look like?
Well, turn Slack and email notifications off for a couple of minutes and read our checklist of UX research plan, and A/B/n testing process needed to build an effective CRO program.
UX research plan
- Data tracking setup audit.
- Event mapping.
- Marketing analytics. Top performing traffic sources and its scalability bottlenecks.
- Keywords and user-intent analysis.
- Top performing creatives and insights for UX.
- Top landing pages.
- Segment analysis.
- ABC analysis.
- Funnel analysis.
- Cohort analysis.
- LTV, usage and transaction frequency.
- Personalization opportunities.
- User journey map.
- User flows.
- Main drop-offs and bottlenecks.
- Behavioral patterns.
- Event correlation and feature usage. Regression analysis.
- CTR analysis.
- Competitor UX analysis.
- Competitor UVP and features analysis.
- Competitor A/B tests, product and website changes.
- User personas. Persona testing.
- “Jobs to be done” research. User tasks research.
- Audit of UVP and it’s perception.
- NPS analysis. Customer satisfaction survey questions.
- First time user experience (FTUX).
- Bounce-rate analysis.
- Relevancy of user intent, keywords and ad messages to landing pages.
- Loading speed analysis and correlation with conversions.
- Screen sizes, cross-browser, cross-device and conversion correlation.
- Onboarding audit.
- AHA moment and conversion to activation analysis.
- Conversion barrier research.
- UX content audit.
- Unanswered user questions.
- Conversion barriers.
- User rejections, fears and concerns.
- Core purchase motivation and triggers research.
- UX heuristic analysis.
- Usability audit.
- UI audit.
- Form analytics.
- UX tests. User testing questions.
- Video session recordings.
- Online polls. Open-ended and closed-ended questions. Poll targeting and triggers.
- User interviews. Respondents recruiting based on data, user poll answers and visitor session recordings.
- Heatmap analysis. Scroll depth, correlation of scroll and funnel progression.
- User feedback analysis.
- Customer support feedback.
- Sales team interview and questionnaires.
- Audit of business model and monetization tactics.
- Potential pricing experiments. Price elasticity.
- Upsell, cross-sell and down-sell opportunities.
- Post-conversion behavior research.
- Thank You page marketing audit. Referral tactics optimization.
- Technical audit.
- QA and bug detection.
Sounds like plenty of homework, right?
Based on our experience of running A/B tests on 127 million of our clients’ users per month, we see that hypotheses without direct interconnection of cause and effect data tend to have 2-10x lower growth and win rates (if you follow cro program best practices listed above).
But how do we actually come up with designs and content for alternative versions?
Based on our experience, depth of UX research has an inverse correlation with the uncertainty of how to design alternative versions. Meaning, if the 57 steps for creating a successful cro program are done right then it’s obvious what and how to test.
A/B/n testing framework
Ok, so let’s assume we already did the research, prioritised a backlog of hypotheses and finally have a CRO action plan. Obviously, the most important thing in implementation of a cro program is to A/B/n test the hypotheses in a most efficient way.
Let’s go through the process as if we were launching a very first experiment.
- Define a macro conversion metric that best describes impact on your revenue growth. We typically define that based on frequency of usage or purchases. For transactional companies like Airbnb or e-commerce stores where users typically make one transaction less than every couple of months, the best metric is average revenue per user (ARPU). For subscriptions or products with long term usage, we define a leading indicator that forecasts LTV, like a 2nd month subscription payment. If you already have a North Star metric, then just choose that.
- Define secondary metrics that should not be dropped like bounce rate, refunds, additional operational costs, specific retention or a usage metric. Such metrics may not necessarily be reflected in short-term revenue but may cause long-term risks.
- Estimate the needed sample sizes and minimal detectable effect of the winning experiments. Define if it’s enough traffic for A/B/n testing or it’s better to go with A/B tests.
- Launch an initial A/A test to check, validate and calibrate the A/B testing tool or in-house traffic split solution and data tracking setups. You can also run a bunch of A/A/B tests if you have sufficient traffic and want to have additional confidence in statistical significance (for example if you want to establish trust with a CRO agency).
- Estimate opportunities for parallel testing where users take part in several experiments at the same time. You may hear that it’s forbidden to test that way in most popular CRO blogs, but companies like Microsoft, Booking, Google, Netflix and LinkedIn do that to run 10,000-50,000 experiments simultaneously.
- Estimate opportunities to cut the time that’s needed for statistical significance like the CUPED method or targeting the test only on users that actually have a different UX (for example if the change is on the 3rd screen of the landing page then only run the test on users who scrolled till the 3rd screen).
- Create an A/B/n testing calendar with approximate estimated times to stop experiments and develop the new ones. Avoid pauses without any live experiments. If we think of growth as a number of experiments then one week without tests means 25% slower monthly growth (and even slower when compounding the decline of each month together).
- We assume that the 57-steps of UX research plan was done and the hypotheses are maniacally prioritized, right?
- Choose a statistical formula that works best for your specific metric, type of dataset and its distribution. Lots of teams just blindly use statistical calculators after reading a bunch of blog posts on A/B tests statistics. Take time to understand the nature of statistical concepts. We recommend the book “Statistical Methods in Online A/B Testing” by Georgi Z. Georgiev as a good foundation for that.
- Prepare an automated dashboard that monitors all needed statistical metrics, sends notifications on significant drops, tracking and splitting issues, and recommends when to stop the test.
- Allocate a dedicated A/B/n test development, QA and analytics team that works on nothing but the experiments. If you don’t feel like doing that or don’t have the resources, read step 64 again – if the whole team is not 100% focused only on growth, it will be inevitably slower. If it’s still hard in terms of resources or it’s hard to hire and build more growth teams you can outsource the A/B test development to a CRO agency. It’s safe, secure as it’s no impact on the actual source code and access to that if done through client-side A/B testing tools like Optimizely and Google Optimize.
- Develop the test and conduct manual QA.
- Set up additional data tracking if any new elements are planned on alternative versions.
- Launch an experiment on a small portion of traffic that’s significant enough to check the correctness of tracking, experiment targeting and help to identify bugs and technical problems.
- Ask the QA team to watch visitor session recordings of the alternative versions to detect bugs that were not found during manual QA or by quantitative metrics. That will also help to uncover the use cases and flows that should be tweaked to polish the hypotheses before the final launch.
- Steps 61-70 should be done every time… and in fewer than 7-14 days to avoid days with no testing.
- It’s time to launch!
- Check the experiment metrics in the dashboard and sit tight until the needed sample size is collected or it’s evident that there is a significant issue or drop, or the experiment is likely to never be significant.
- When it looks like it’s time to stop the test, check the outliers and define a method to clean them up if any. Visualize the transactions on the plot to visually understand the nature of the data set. This will help to choose the best way of dealing with outliers like filtering with 3 standart deviation, defining the theshold or replacing transaction volume to average numbers.
- Time to stop the test!
- Conduct post-test analysis to specifically understand why the test won, lost or made no impact by looking at micro-conversions and segments that were impacted by the alternative version. This step is critical to CRO research and learning things for the next hypotheses or creating a tweaked version of the current one. This step makes sure that the experiments actually had no mistakes as you’ll get more data than in the initial pre-launch.
- Check personalization opportunities by looking at separate segments that have statistically valid growth.
- Choose a way to estimate the actual long term impact after implementation. You can check cohorts of A and B after 1,2 and 3 months after stopping the experiment. Or implement the changes on 90% of traffic instead of 100%. Define the amount of traffic and frequency of rolling up new versions based on the needed sample size for significance. Another way to do that is to repeat the winning experiment before implementation or to run B/A some time after implementation. Repeatability of experimental results is a main feature of true scientific knowledge!
- It’s time to implement the winning version and repeat the process time and time again!
To sum up, these 81 CRO program steps could dramatically increase the growth and success rate of your experiments if done right. If you’re launching a CRO program for the first time or for a new product, then it’s critical to go through all of the steps.
Product managers often don’t have the resources, time, patience or expertise to execute that to a full extent, leading to hypotheses with a bunch of unknowns, like no exact data on cause and effect, no exact quantitative prioritisation, etc.
When you guess the biggest problem, then guess the reasons for that, and afterwards assume the solution, then the probability of winning is lower than when you have the exact data on this.
So “Arriving at hypotheses, scientifically” as Michal said is the core thing that defines an effective CRO program.
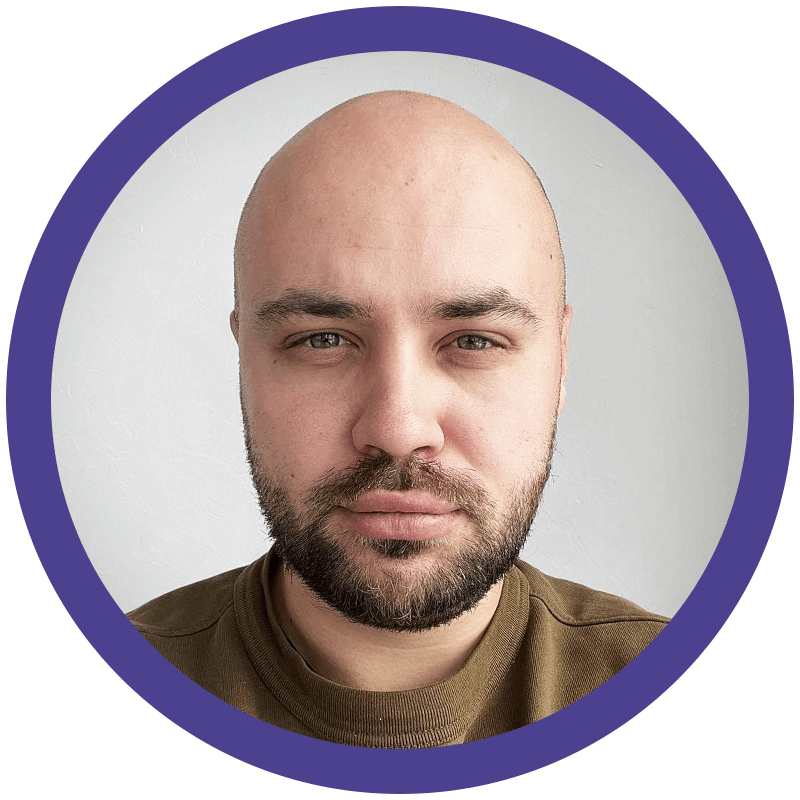
Glib Hodorovskiy, co-founder Conversionrate.store
Glib Hodorovskiy is a CRO strategist that conducted thousands of experiments on hundreds million users.
He meditated 3000+ hours, teaches mindfulness and is passionate about neuroscience of attention and decision making.